Introduction
Many organizations today are struggling with the question of how to handle their data. A number of things are important in order to gain real added value from this data. The starting point must be that the data will work for the organization and not the organization for the data. With the right data strategy, much more can be achieved than organizations currently think.
In order to achieve a good data strategy, an organization needs to ask itself three essential questions:
- What does the organization want to achieve in the future?
- What information do you need for these objectives?
- How should the data work for you?
Question 1: What does the organization want to achieve in the future?
Without an answer to this question, it is difficult, if not impossible, to formulate a good data strategy. More and more information is available and there are more and more possibilities to collect data. In addition to the classic dashboard that provides insight into the current status, it is now also possible to make predictions using algorithms (Machine Learning/Artificial Intelligence).
In order not to get lost in the jungle of possibilities or take wrong turns, it is important that the data strategy supports the organization’s strategy. Where you as an organization want to go in the future, determines how you deal with the data.
Core elements to answer this question are:
-
What do you want to be able to offer customers as an organization, what is your future revenue model?
- How do you want to work in the future, what do you want to automate and what is better to be done by humans?
- How do you see the role of the organization in the chain in the future?
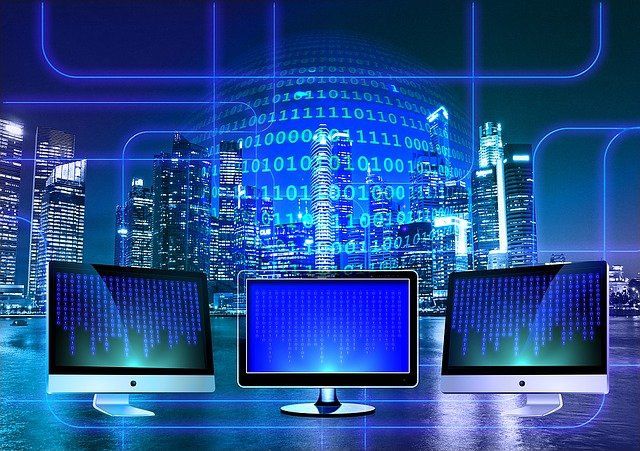
Question 2. What information do you need for the objectives?
It is important to understand what data you need to achieve your goals. With this input, you can identify the white spots, the information that is not available. You can proceed with the right target: how can the organization fill in these white spots?
For example, a transport company may decide to monitor the rides so that a white spot can be filled in and they can optimize the driving schedule.
In addition to filling in white spots, it is very important to set up the data in such a way that it works and remains manageable for current and future processes. In this process, it is best not to distinguish between internal or external data. Setting up the data management process independently of the owner, not only makes it more future proof, but it also creates the flexibility to quickly add new data sources. Effective data management allows the organization to focus on the exploitation of the data.
Core elements to answer are:
- What information do you need and what are the white spots?
- Who is responsible for the data and how will you as an organization make decisions about the data?
- Which data is your own and which data will you get externally?
- Do you want to share (part of) the data with other (chain partners/customers)?
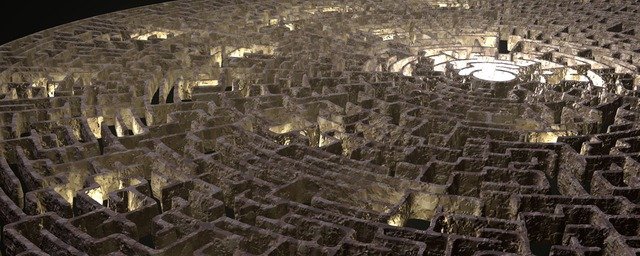
Question 3. How should the data work for you?
Because there is so much data, it is important to know when do you want to do what with the data. A lot of organizations spend a lot of energy and cost setting up so-called data-lakes and large data warehouses in which they run all the data. However, when converting to applications, the insight that often follows is that a lot of data has been collected but just not the data that is needed. On the other hand, the failure of an AI project is often dedicated to a lack of data, so that the correct predictions cannot be made.
The most important way to not end-up in the pitfall of too much/too little data, is to answer the question up front: What should the data do for the organization?
In order to be able to answer this question properly, it is useful to create a pragmatic agenda/roadmap. Collect as an organization the necessary new ways of working that you want to do in the core processes and plot them according to the urgency in the agenda/roadmap.
In this way, you ensure that the new data applications get a role in the knowledge processes and you can immediately start to realize them. With a good demarcation, this realization does not have to take long. Cycles of 6 weeks or 12 weeks are possible.
Core elements to answer this question are:
- How do I want to redesign my core processes?
- What is the urgency and priority of the new applications?
- How do I ensure a good demarcation?
Practical tips for a good data strategy
To make data work for you in a practical way, a number of principles are best followed:
- Use a layered approach that separates the following things:
- Sources layer: with the sources from which the data is retrieved
- Data layer: where the data comes together to feed the algorithms
- Algorithm layer: where the data is applied in new ways to make analyses and predictions
- Orchestration layer where everything comes together so that users can work with it on a daily basis
- Provide scalable and flexible processes to manage and adjust data flows.
- Don’t Panic: There is always a way or an alternative to obtaining the desired data in order to realize the new applications with data.
- Fast, smaller projects often generate more value. Please note that an application is only valuable if it can be used on a daily basis.
Recent Posts
Business
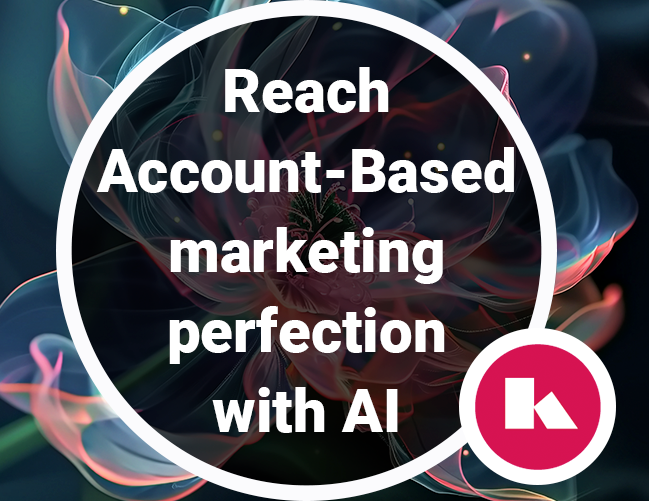
Reach Account-Based Marketing perfection with AI
While Account-Based Marketing (ABM) may appear to be a natural evolution of targeted marketing, there’s much more...
A.I.
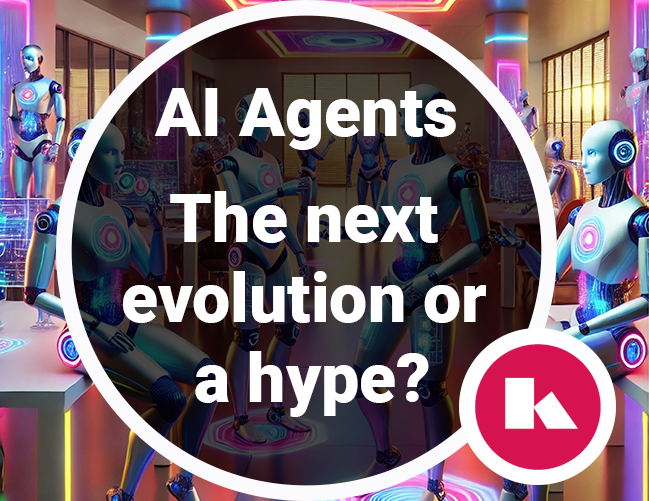
AI Agents, the logical evolution that is hyped
Agents are the latest buzzword in AI, often positioned as the next evolution beyond Generative AI (GenAI). The concept...
Company
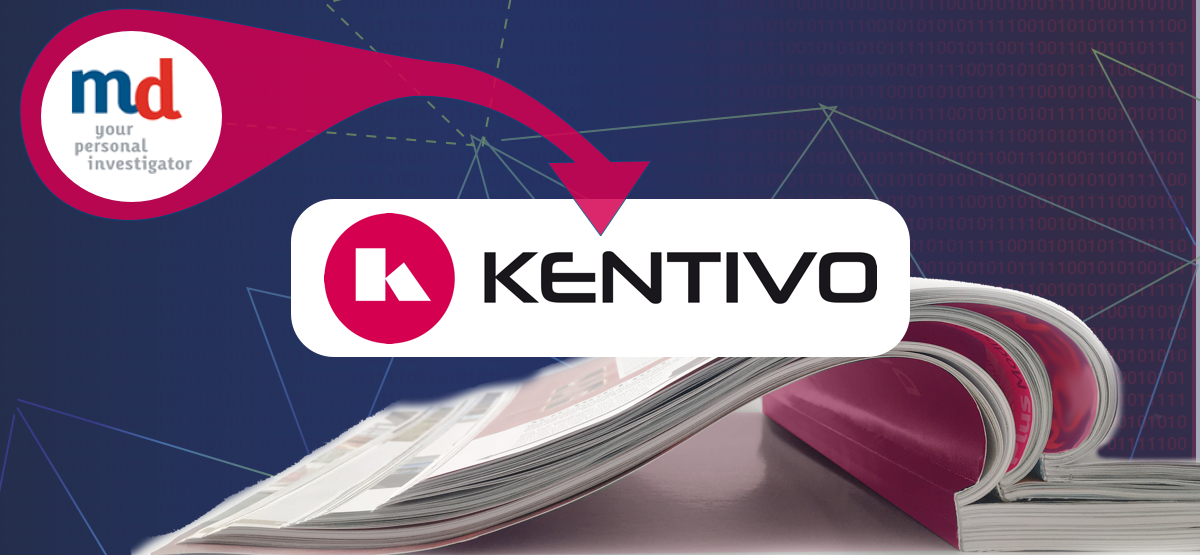
Kentivo Group acquires Media Digitaal B.V. (MDInfo)
The Kentivo Group of companies is delighted to announce the acquisition of MediaDigitaal B.V. in Amsterdam, The...