TeamSketcher
While working with our customers, we noticed the same need appearing in many companies, regardless of the business they are in. Sooner or later, an increasing number of them run into a problem of finding the right person to perform a certain (part of a) project. Whether it’s a business expansion to a new country, or new, specific expertise is needed for a project, the fast-paced business world often requires making new connections in less known spheres, and fast! Even if all the important players are already there, very often there is still an issue of how to use the best, considering the time and financial constraints. We see the number of variables and their potential combinations is just getting too large to keep in mind while doing the decision making. Being able to develop a team that works with high effectiveness and minimum resource waste can make or break a company. If this sounds familiar this post is for you. This is exactly is where machine learning can help you!
“The Best Team For The Job Is Somewhere Out There. Let Machine Learning help you find it!”
post is for you. This is exactly is where machine learning can help you!
Within Kentivo we developed a tool, TeamSketcher, with several functionalities. From selecting individuals to building entire teams, based on information available, either internally (company sources) or externally (online sources). Let’s name two examples:
Managing workflow capacity
For knowledge driven organization, the core resources are the people and their skills. Machine Learning can help you with the proper selection of individuals and teams for interdisciplinary projects. Not only with giving advice on resources to allocate for new projects, but also provide insight in potential impacts on other projects, which will definitely help with planning new projects
Creating an international team
It offers a fast, interactive, and smart way to present the company and its employees, to show a potential client the talent at your disposal, but also to enable on the spot, in depth overview of what the possibilities are when negotiating future collaborations.
How does it work?
An algorithm starts with collecting important information that needs be considered- such as geographical location, expertise, seniority, previous experiences. The user decides on the variables of importance. Once the algorithm is fed these contingencies, it sieves through the database, making sure to find all the matches. The first output is a list of potential candidates, that then gets pruned and refined. At this stage the algorithm is leaving a very broad margin, not limiting the search outcomes too much, thus giving a user free space for finesse, to tailor the search to specific needs before the final selection. This also gives the opportunity to play around and see the outcome change if a search term is added or removed, or if a value is altered per unit of measurement. Our clients report this to be a very valuable aspect of the process. The outcome of their own, mental search, which depends on personal knowledge and experience, is compared, and then combined, with the impartial knowledge of the algorithm. Usually there is an “oh wow, I didn’t think of that” moment.
In the second step, the TeamSketcher gives an opportunity to combine the individuals and build teams, depending on relevant criteria. An unlimited number of skills can be chosen, and the algorithm will always make sure to respect the main criterion- resourcefulness. While humans tend to fall prey to certain cognitive misconceptions during decision making- familiarity, primacy effect, or anchoring, to name a few, the collaboration with Machine Learning ensures building a team efficiently, minimizing the waste of talent, time, money, and effort.
As stated in the beginning, sometimes the variables are just too large. The benefit of using Machine learning is that it has the power to look beyond and above just a single project for which the team is being built. The algorithm can handle huge amount of data and variables and can help to make decisions, potentially avoiding over- or under- staffing, using, if available, even the information on the soft skills, personal career plans and motivations, for a well-rounded team that can work together.
Recent Posts
Business
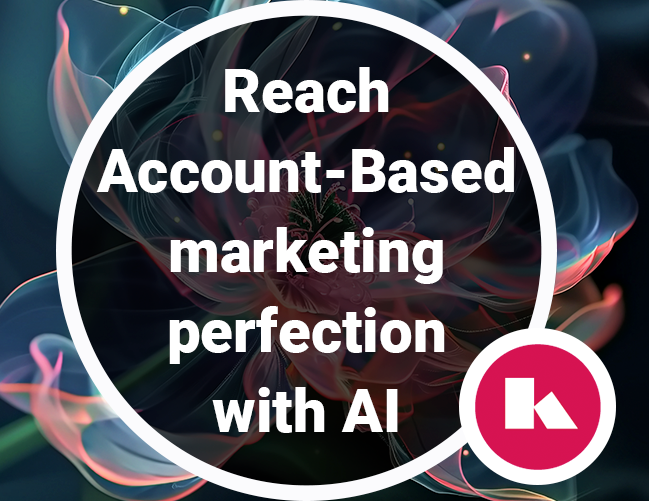
Reach Account-Based Marketing perfection with AI
While Account-Based Marketing (ABM) may appear to be a natural evolution of targeted marketing, there’s much more...
A.I.
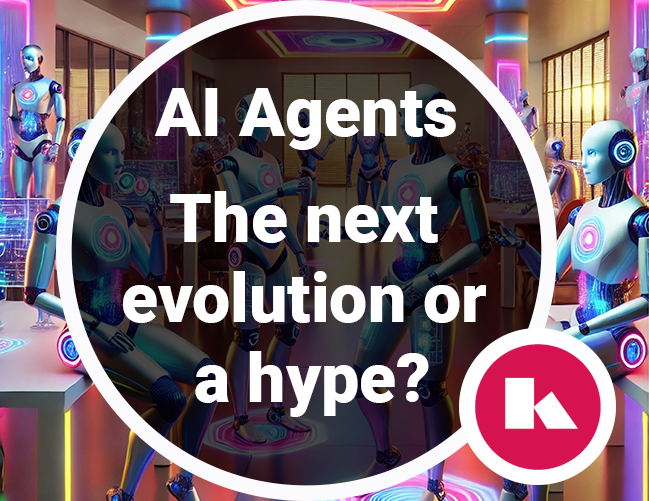
AI Agents, the logical evolution that is hyped
Agents are the latest buzzword in AI, often positioned as the next evolution beyond Generative AI (GenAI). The concept...
Company
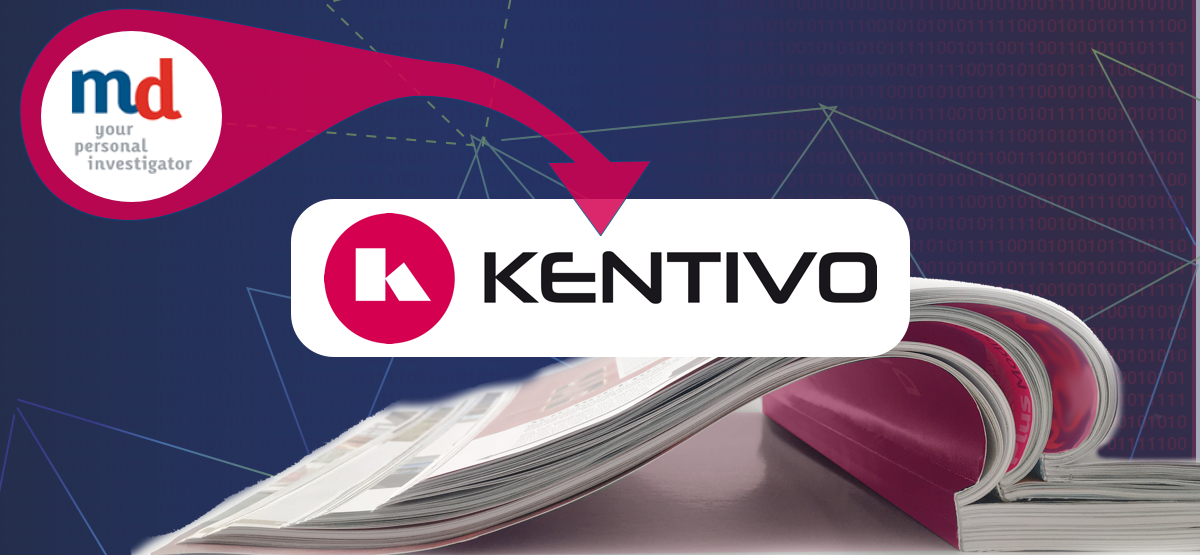
Kentivo Group acquires Media Digitaal B.V. (MDInfo)
The Kentivo Group of companies is delighted to announce the acquisition of MediaDigitaal B.V. in Amsterdam, The...